Know Your Customer
How do you predict your customers' actions and create a customer retention plan?
​
Let's create one for a telecommunications company using an IBM dataset.
I love my telecom service and will stay!
​
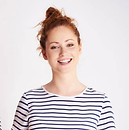
73%
Customers Did Not Leave
(a.k.a Not Churn)
I don't like my telecom service and want to leave.
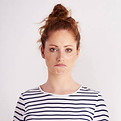
27%
Customers Left in a Month
(a.k.a Churn)
​
Factors that may increase or decrease the probability of customers leaving.
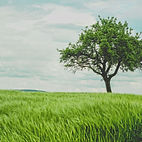
Paperless Billing
Customers either have paper bills or electronic ones.
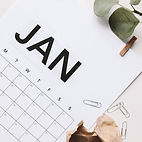
Monthly Charges
How much charged to a customer every month.
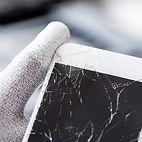
Device Protection
If customers choose device protection.

Online Security
If customers protect themselves online.
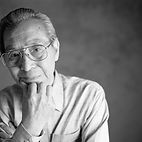
Senior Citizen
If a customer is a senior citizen.
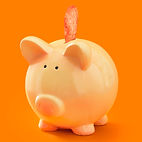
Total Charges
Total money charged to a customer.
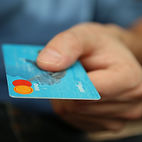
Automatic Payment
Customers pay their bills automatically either through bank or credit card.

Tech Support
If customers have technical support.

Multiple Lines
If a customer has multiple phone lines.
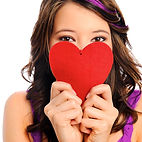
Tenure
How many months a customer has stayed and used service.
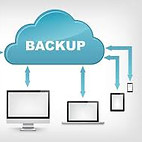
Online Backup
If customers back up their data online.

Yearly Contract
If customers have a one year contract or more, not month to month.
Let's see these probabilities with a Linear Probability Model.
Data in One Month Taken from a Linear Probability Model with P Value of .05.
P Values indicate that these factors are significant and meaningful for the telecom company to consider.
But what factors does a Decision Tree tell us is important (in order)?
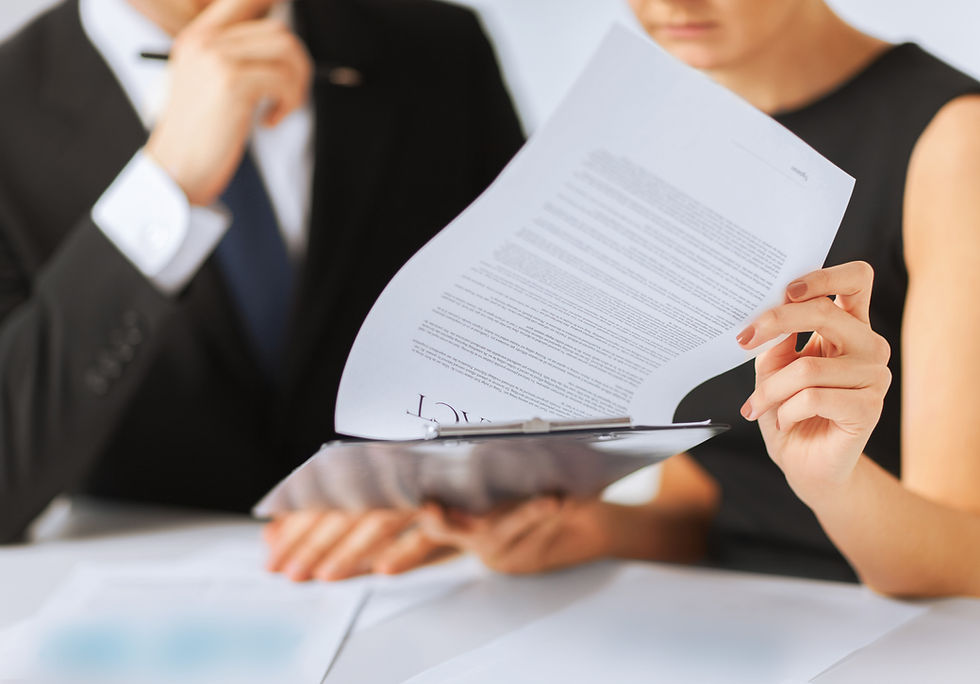
Churn Probability of 7% (Lowest)

*Not significant in LPM
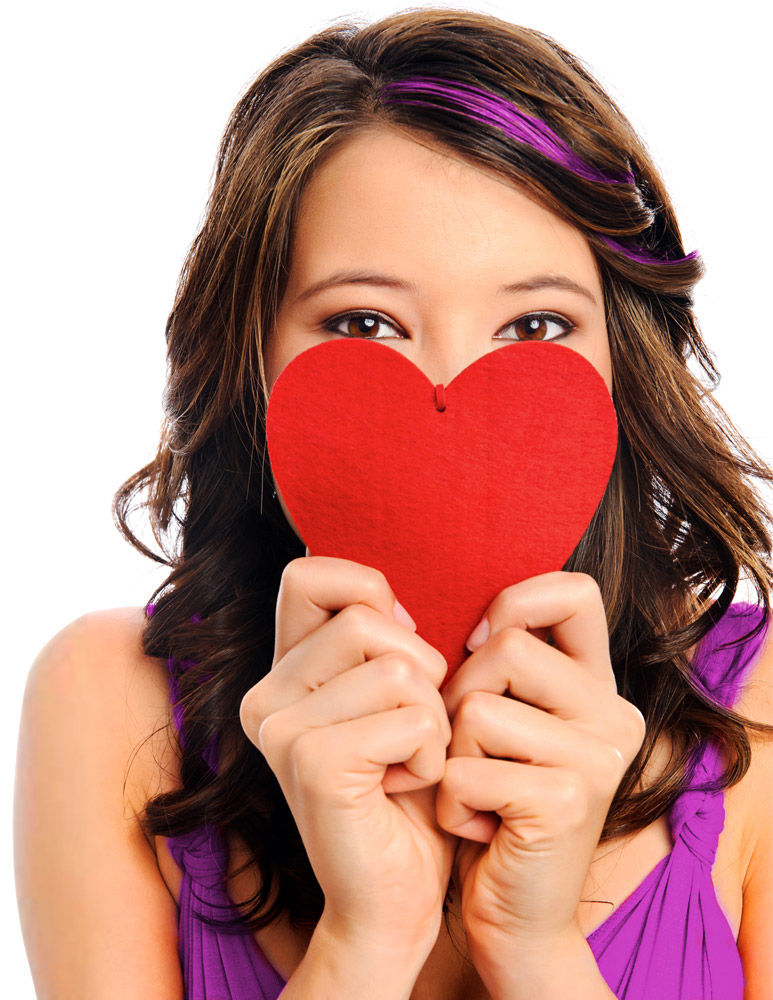
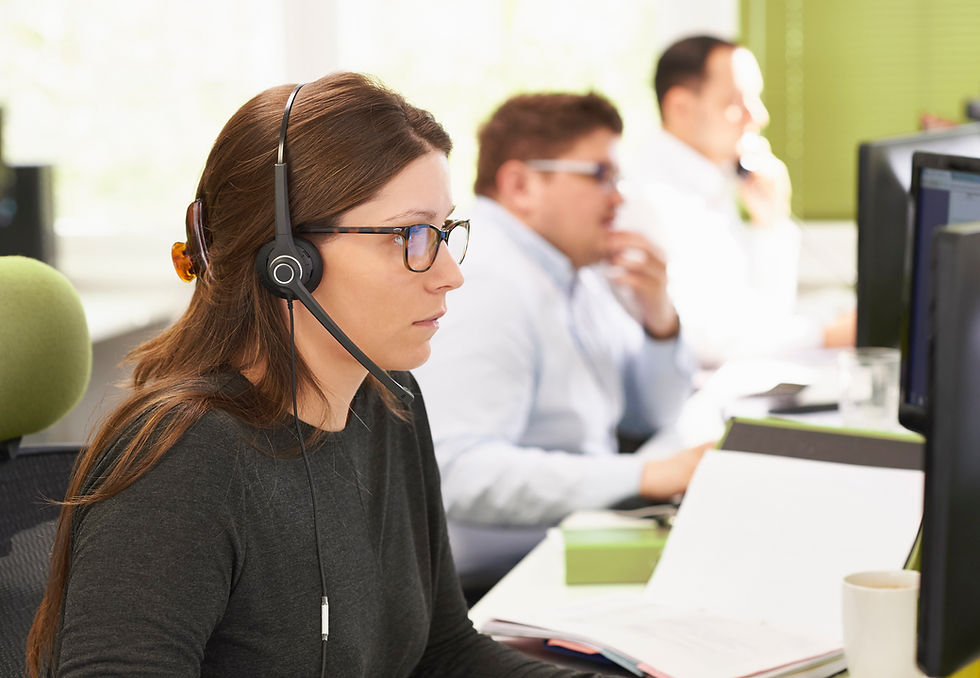
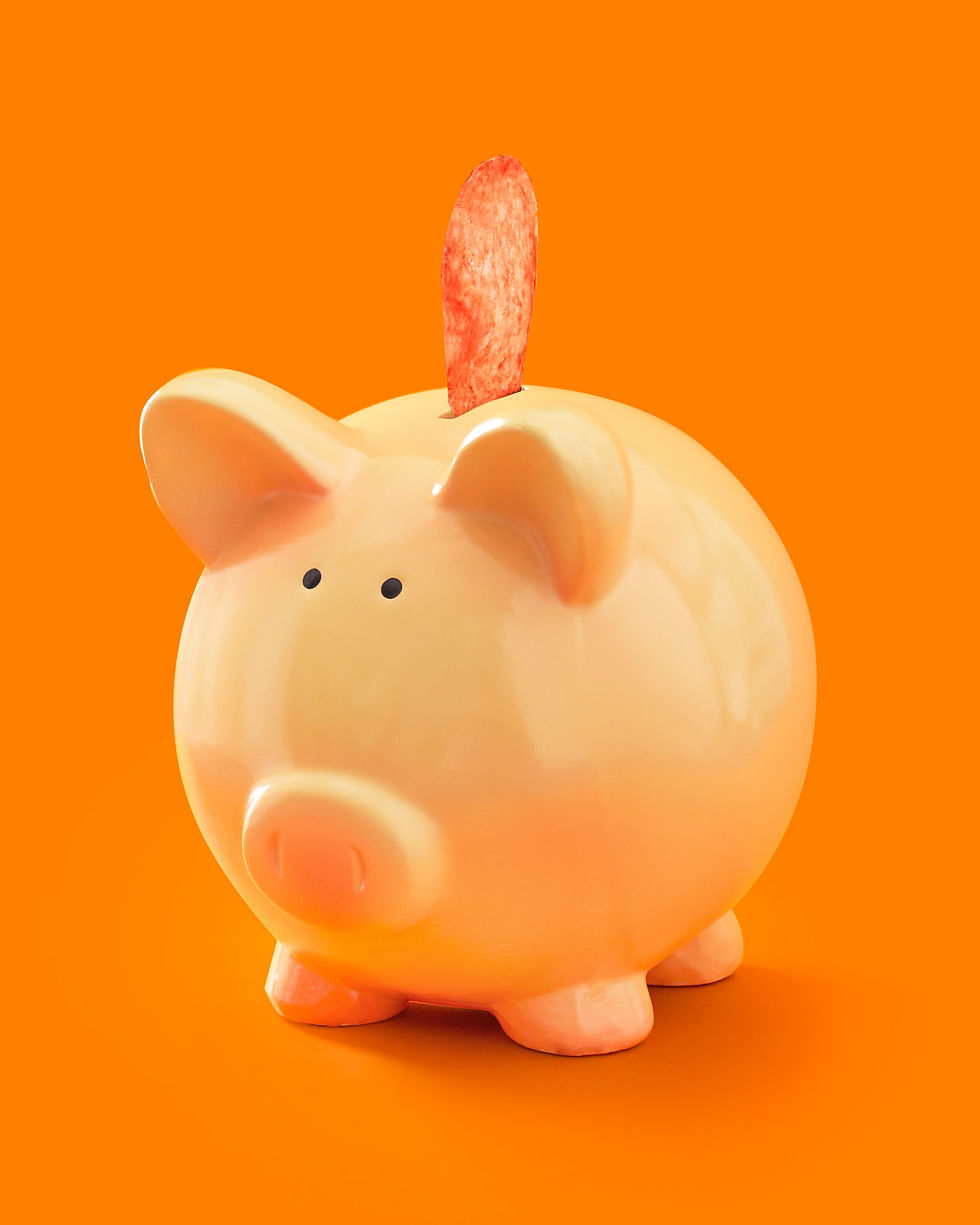

So according to these factors what kind of customers might we have here?
The Happy
Customer
Has a yearly contract with automatic payment.
Enjoys relevant services
like tech support, online
security, online backup,
& device protection and
might be willing to pay extra.
Has a relatively longer tenure compared to others.
Happy Customer
The
Customer
Who May Leave
Senior Citizen
Has paperless billing but
might prefer paper instead.
Has multiple lines but might not need it.
Not willing to pay for extra monthly charges.
Customer Needs
Better Service
Retention Plan: Be Convenient and Relevant
Yearly Contracts
Strive to make current month to month customers sign yearly contracts. This could be done by lowering monthly charges, which could further entice customers to stay. Furthermore, a loyalty rewards program could increase tenure or length of contracts.
Switch Customers to
Relevant Services
Target customers who have multiple lines who might not need them anymore and provide special discounts or offers for tech support, online security, online backup or device protection. Customers may be willing to pay more and stay if they have relevant services that they find useful.
Paper Billing
By Default
It will make it easier for customers who prefer paper. If the reason for paper is remembering to pay on time, suggest they enroll in automatic payment to provide extra convenience. Those who want to go paperless should still have the option.
Survey Senior Citizens
A survey on the phone could provide more information on what seniors find relevant and convenient from your service.
Notes
-
Decision Tree: since the output variable is churn/not churn, we can use supervised learning to predict the probability of a current customer churning or not. We can also look at the leaf nodes and “follow up the tree” to create a basic customer profile.
-
CP – .001
-
Minsplit Factor – 80
-
These settings have been established to create an accurate tree that is pruned enough to create an understandable customer profile (see Classification Rate and CP Factor),
-
-
Linear Probability Model: this might not be the best model because the dependent variable should be continuous and, in our problem, the dependent variable is not. This is due to the dependent variable being either 1 or 0 for Churn/Not Churn. However, a LPM usually (though not guaranteed) yields consistent results.
-
We will set a common P-Value of less than .05 to find out whether any of the coefficients of the independent variables are significant and meaningful and are not just up to chance.
-
From the significant coefficients, we can see which independent variables are most probable to increase or decrease churn.
-
All data will have to be converted into numerical or binary data (1 if Yes and 0 if No).
-
In the cases of Gender, it will be converted into 1 for Male and 0 for Female.
-
For Internet Service, it will be converted into 1 for Fiber Optic and 0 for Other.
-
For Contract, it will be converted into 1 for Yearly Contract or 0 for Other.
-
For Payment Method, it will be converted into 1 for Automatic Payment Method or 0 for Not Automatic.
-
-
When a customer has phone service with Telco (binary variable) they have a 15.63% less probability of churning (the decision tree though does not list phone service anywhere in the tree).
-
In terms of prediction for LPM, it yielded a slightly low Multiple R-squared = 0.2803. However, for this set of data this could be acceptable due to a variability and unpredictability in customer’s decisions to churn. There could also be other outside factors not included in the data set (like region, etc.).
-
Checking Assumptions
-
Linearity: for there to be linearity the x variables need to be continuous in most cases, so we cannot assume perfect linearity when we used non-continuous data.
-
Exogeneity: there could be other factors such as region, # of service interruptions, switching due to faster internet providers, etc. which could affect the reasons why customers churn.
-
Lack of Multicollinearity: (see here) the x variables do not seem to be highly correlated with one another.
-
Homoscedasticity: because it is a linear probability model there will be some heteroscedasticity.
-
-
-
K-Means Clustering: since we have mixed continuous and binary data, K-Means clustering might not be an ideal model due to the Euclidean distance measure. However, we can cluster the top five or six independent variables in the decision tree with the significant coefficients in the linear regression which we believe are related to Y (churn) and compare different customer groups or profiles.
-
We will choose 7 clusters to keep a manageable overview of potential customer profiles while also maintaining a higher cohesion, or low Within−Cluster SSE (see here).
-
It is important to note that phone service was above average in all clusters so it is hard to determine if it is specific to any type of customer.
-
​
Website design and layout by Graam Liu
​